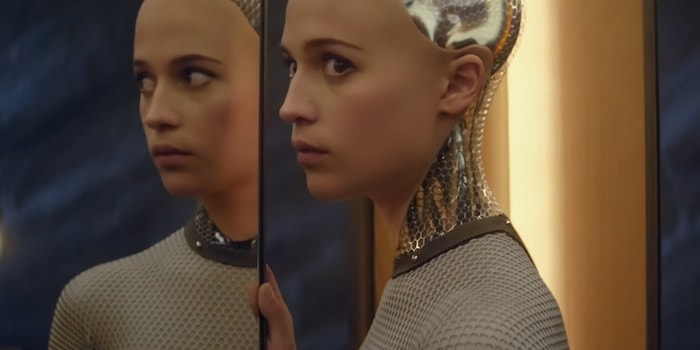
Artificial intelligence - when is it considered intelligent?
Siri, Google Assistant, Cortana or Alexa make your life easier. The camera and software on your smartphone calculate the optimum image for you. Artificial intelligence (AI) can do more and more. At what point do we have to be afraid that artificial intelligence will overtake us?
The idea of artificial intelligence is very old. Greek mythology already mentions intelligent robots. In one depiction, Talos is a bronze, automatic giant. His task is to protect Europa, Zeus' lover, on Crete. To protect her, he throws stones at approaching ships. Should a crew make it ashore anyway, the giant heats up so that the invaders get scared and flee. If they are not deterred, he kills them by embracing them. Incidentally, in the story, Talos was killed by the Argonauts - the band of heroes who help Iason in his search for the Golden Fleece.
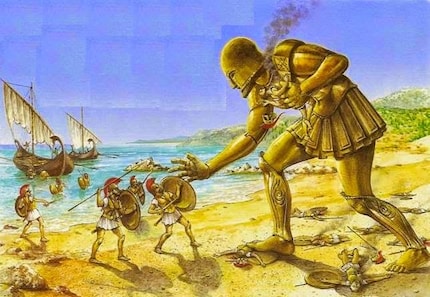
Source: //greekmythology.wikia.com/wiki/Talos
Luckily, we no longer have to row across the seas like the Argonauts to enjoy the sight of AI. Talos already possessed a central characteristic of human intelligence: it adapted its behaviour depending on the stage of the invasion, it was capable of learning. Of course, the ability to learn is only one characteristic of human intelligence.
Let's take a look at a more recent example from science fiction. In the film "Ex Machina", the main character Caleb is supposed to subject the android Ava to a Turing test. I don't want to spoil too much for those who haven't seen the film yet. But it turns out that Ava is a calculating being. She has many characteristics of human thinking: problem solving, learning, reasoning, perception and language use.
Problem solving
Problem solving can be defined as systematically trying different actions to achieve a defined goal. Problem-solving methods in AI can be categorised into general methods and special methods. A specialised method has been developed specifically for a particular challenge. General methods can be applied to multiple challenges.
When solving problems, the AI is programmed from scratch. The knowledge is therefore not self-acquired, but comes second-hand through programming. AI focussing on problem solving was particularly relevant at the beginning of AI research. But more on this in another article.
Learning
There are various approaches to how an AI can learn. The simplest approach is trial and error. Here, the AI pursues various approaches. In doing so, it consciously accepts that one approach may be wrong. If one then works, it is used again and again in the same situation. This approach is called Rote Learning.
Another approach is machine learning or its further development, deep learning. Machine learning algorithms use their calculations to learn directly from data without relying on predefined programming. In contrast to raw learning, the AI is intended to carry out actions without explicit programming. The algorithms improve their performance when the amount of relevant data for learning increases. This is why machine learning and deep learning have made great progress with big data in recent years.
One example of machine learning is Netflix's recommendation function. Based on the information collected on user behaviour, the algorithm calculates what else the user might like.
Google's Alpha Go is a good example of deep learning. The software, which masters the complex game Go, learns by competing against human Go players. This works so well that Alpha Go has already beaten top players. In contrast to machine learning, Alpha Go learns everything itself thanks to deep learning algorithms. By definition, machine learning still needs to be assisted here and there.
A distinction must be made between learning and reasoning. In learning, AI relies on data; in reasoning, laws are crucial for AI.
Inference
Inferential thinking is used to draw conclusions. Conclusions can be either deductive, inductive or abductive. In deduction, the consequence is inferred from a premise and a rule. Induction involves drawing conclusions from the premise and consequence to a rule, while abduction involves drawing conclusions from the rule and consequence to the premise.
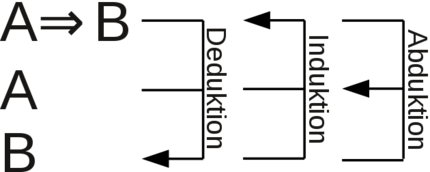
Source: //de.wikipedia.org/wiki/Schlussfolgerung
Example of deduction
Livia is either at work or at school (rule)
She is not at work (premise)
She is therefore at school (consequence)
Example of induction
Livia is not at work (premise)
She is at school (consequence)
So she is either at work or at school (rule)
Example of abduction
Livia is either at work or at school (rule)
She is at school (consequence)
She is therefore not at work (premise)
Induction and abduction are logical conclusions. Only deduction is absolutely necessary. Deductive reasoning is also at home in mathematics and logic.
These examples are, of course, highly simplified. However, they illustrate the difference between deductive, inductive and abductive reasoning very well. Artificial intelligence is already very strong in deductive reasoning. However, true reasoning involves more than simply drawing conclusions on the basis of rules and premises. It involves drawing conclusions that are relevant to the solution of a certain task or situation. Human agency requires all forms of reasoning.
An example of deductive reasoning is AI that can perform complex mathematical tasks. Personally, I am not aware of any example of AI that uses deductive or inductive reasoning. In most cases, this is machine learning again. If you have a real-life example of AI that uses inductive or abductive reasoning, please let me know in the comments.
Perception
During perception, the environment is scanned by various sensors. The objects and subjects located in a predefined space are then displayed and perceived in their spatial relationship to each other. This allows actions to be performed in the room. This also includes walking and grasping, for example.
An example of perception in AI are robotic arms that assemble an Ikea chair. Various sensors scan the environment. The robotic arms recognise where the parts are and can pick them up and put them together. The artificial arms also have pressure sensors to estimate how hard they need to push in dowels, for example.
Language
Language is a sign system that derives its meaning from conventions. It can therefore not only be reduced to spoken language. In this respect, traffic signs, for example, can also be regarded as language. Human language, however, is capable of forming countless sentences. Words can have double meanings and be pronounced differently due to dialects, accents or speech impediments.
There are plenty of examples of AI and language. There are the voice assistants that accompany us on our smartphones and, increasingly, the smart speakers in our smart homes.
Complex matter
Human intelligence is much more complex than initially assumed. It takes a lot for artificial intelligence to compete with human intelligence. Imitating one or even two components of human intelligence seems possible. But language, perception, problem solving, reasoning and learning at the same time? That seems very complex. An android like Ava will probably remain science fiction for the next few years.
Perhaps that's a somewhat bold hypothesis. A breakthrough could suddenly happen very quickly. But in order to look into the future, we first need to visualise what has happened so far. I'll be writing about how artificial intelligence has developed so far in another article soon.
19 people like this article


From big data to big brother, Cyborgs to Sci-Fi. All aspects of technology and society fascinate me.